What's limiting AI in transforming research and drug discovery? And how can we solve these problems?
.webp)
Lifebit
December 2023
Author: Hannah Gaimster, PhD
Contributors: Hadley E. Sheppard, PhD and Amanda White
Introduction to health data, drug discovery and AI
Healthcare is one of the most data-rich industries. This health data is increasingly used in research where it is needed to develop effective new disease treatments in drug discovery.
However, due to the volume of data that needs to be analyzed, researchers can struggle to utilize this data effectively. Artificial intelligence (AI), a field that uses robust datasets and computer science to facilitate problem-solving, can help researchers accelerate this process.
The promise of AI- vast potential but limited results so far
AI promises a revolution for drug discovery, allowing computer systems and software to perform tasks that typically require human intelligence. While there have been success stories, investments in technology to elevate data and analytics have not yet achieved the profound impact required by the pharmaceutical sector.
This article discusses the critical challenges and barriers to incorporating AI into research and drug discovery and how these challenges are being overcome.
Challenge: Using AI on health data requires maximum data security and privacy
Large-scale health data data is highly sensitive, so software to access and analyze it requires a security-by-design approach.
Solution: Federated data analysis
Data federation addresses the issue of accessing data while ensuring its security.
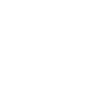
Featured resource: Discover our ultimate guide to federation
This software process allows multiple databases to collaborate as a single unit and helps keep patient health data safe within the proper jurisdictional boundaries. A federated platform brings the analysis of authorized users to the data so that it does not have to be downloaded, copied or moved from its original location, activities which are time-consuming, computationally inefficient and costly. A federated approach, can leverage scalable integrations to wherever the data resides, facilitate secure access to data and the latest analytic algorithms, and facilitate seamless collaboration amongst researchers.
Challenge: Leveraging AI requires large amounts of data to be accessed to train models
Access to vast amounts of data is necessary to train models to use in AI successfully.
Solution: Scalable cloud infrastructure combined with federation
When dealing with large volumes of patient data, it is crucial to have access to sufficient computational resources. Furthermore, a reliable database infrastructure and a scalable platform are necessary to process and analyze the data effectively. As a result, there has been a growing trend in healthcare data analysis towards utilizing commercial cloud infrastructure, which offers unparalleled flexibility. Due to its elastic nature, cloud computing allows researchers to only pay for the specific resources required to ensure optimum value for money. Researchers can securely link datasets without moving data when cloud infrastructure is combined with federated data analysis.
Challenge: Non-interoperable data provided via analysis platforms with poor usability
Even if researchers can securely access disparate datasets for AI via federation, it will be essential to ensure this data is immediately ready to be combined for analysis via an intuitive and easy-to-use platform. In particular, researchers and clinicians without a data science background may be disadvantaged in using AI and analytical tools that require coding.
Solution: In-house data harmonization is provided via low/no code and user-friendly platforms
Platforms are now available with automated harmonization capabilities to standardize the data through state-of-the-art methods. Data access and analysis software that offers advanced features, such as end-to-end data visualization and reporting, can make it easier for researchers and healthcare providers to gain novel insights from the data and AI.
Challenge: Mitigation of bias in AI
It will also be crucial to ensure the ethical use and mitigation of bias of AI in patient/participant data analysis and for research. An area where this is particularly important is in datasets where diversity is limited. Since the first human genome sequencing, most genetic associations with disease studies have been performed in people with European ancestry. This presents the problem where these datasets only represent one group of people and lack representation of other populations. Only having data from one population group in research studies can inhibit building correct models for AI and forming complete insights.
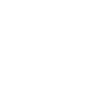
Featured resource: Catch up on our recent webinar on Data Diversity in Genomics
Solution: Limited data diversity can be addressed by securely accessing global cohorts via federation
Recently, experts, organizations, companies and research groups worldwide are driving change to champion diverse and inclusive health data for research. For example, biotech companies, such as Gen-t in Brazil and Omica.bio in Mexico, aim to sequence the Latin American population, a historically underrepresented group in genomic and health studies. Tackling this issue to close the gap in data diversity is needed especially as AI develops.
An example is training AI models to detect skin cancer better using diverse skin colors, instead of lighter skin tones. By using a federated platform to access data from global cohorts securely, this will ensure a more complete dataset to lead to accurate and unbiased medical insights.
Summary
AI can help integrate and analyze enormous and intricate health datasets. However, the critical issues of data access and security, data harmonization compute power, and user-friendly analysis platforms and datasets with limited diversity must be addressed. Solutions including federated data analysis, automation of data standardization cloud computing, end-to-end platforms, and access to diverse global data will help overcome these challenges. These solutions can improve patient outcomes by strengthening diagnostic, treatment, and preventative methods.
About Lifebit
Lifebit provides health data solutions for clients, including Genomics England, Boehringer Ingelheim, Flatiron Health and more, to help researchers transform data into discoveries.