
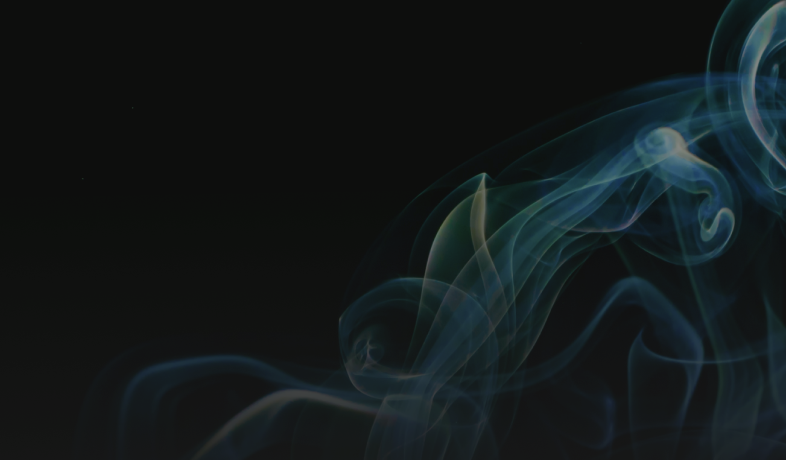
HotNet2 Algorithm: Detecting Cancer Genes & Disease with Precision
Lifebit
Why study cancer heterogeneity? Introducing HotNet2 to support your efforts.
In 2018, 18.1M people across the globe were diagnosed with cancer and 9.4M patients died of their disease.
1 in 2 people will be diagnosed with cancer during their lifetime, regardless of gender. Take a moment to process that.
To make matters worse, close to 50% of cancers are diagnosed in the later stages of development, which unquestionably negatively impacts the treatment outcome. Case in point – ovarian cancer: 90% of women diagnosed with the earliest stage of the disease are still alive 5 years later, but this number drops to 4% when the cancer is diagnosed at the latest stage.
The main reason why late diagnosis leads to less effective treatment is that cancer is a highly dynamic disease which becomes more heterogeneous over time. Not only that, we classify cancer as one disease based on the tissue or the location in which the cancer originates when, in fact, cancers such as ovarian cancer are driven by different mechanisms in different patients. Case-in-point – in Diffuse Large B Cell Lymphoma (DLBCL), that, until recently, was regarded as one cancer type, researchers identified some 150 cancer driver genes and can be sub-classified into two very different types of B-cell lymphoma.
As such, the heterogeneous nature of cancer has enormous implications on the way we treat patients, and challenges the one-size-fits-all treatment paradigm, which some argue may do more harm than good. The recent rise of truly personalised cancer treatments (i.e. cancer vaccines, immuno-oncology and CAR-T therapies) is our best bet to increase patient survival and offer lasting cures. For these treatments to work, understanding and profiling cancer heterogeneity is key.
Cancer heterogeneity
Next-generation sequencing has enabled researchers to shed more light into tumour heterogeneity, establishing that cancer is heterogeneous both within a patient tumour (Figure 1) and across cancer types (see previous DLBCL manuscript). More specifically, scientists have revealed that there are regions within the same tumor that have tumor cells with different genetic mutations and behave differently. Furthermore, it was shown that there are more genetic differences than similarities between biopsies – only about ⅓ of the identified mutations were consistent across all biopsies taken from a single patient.
To make matters worse, pan-cancer analyses have also shown extensive mutational heterogeneity across most cancer types, but only a handful of significantly mutated genes are identified since most exist in only a small number of samples. This is referred to as the long-tail phenomenon, an occurrence which complicates the discovery of significantly mutated pathways: mutated genes that are responsible for causing and driving the cancer (cancer driver-genes) may be indistinguishable from genes containing random mutations that accumulate as a result of cancer progression. If we fail to characterise genes in the long tail, we are losing out on a lot of valuable information for future personalised cancer treatments.
Tackling cancer heterogeneity and identifying cancer driver-genes with HotNet2
So, how can we do better? We need to leverage the mountains of whole-genome and whole-exome sequencing data to paint a more detailed picture of the heterogeneity of somatic aberrations in cancer, including long tail genes. That is easier said than done, as most algorithms that identify mutations in cancer only return the most commonly mutated genes, and tend to overlook rarely mutated cancer driver-genes.
HotNet2, an algorithm developed by Medley Genomics co-founder, Dr. Ben Raphael, and his research group, presents an innovative solution to the problem. HotNet2 detects disease candidate genes by combining biological information (a gene’s mutation) with topological network information (a gene’s topology). It is able to assess the complex genomic heterogeneous landscape across patient cohorts, including long tail genes, by building significantly mutated gene subnetworks based on mutational frequencies and known interaction networks. It was first used in pan-cancer network analyses to identify novel cancer driver-genes using data from the publicly available Cancer Genome Atlas (TCGA), establishing it as a very prominent method to investigate new diagnostic and therapeutic opportunities across cancer types.
Revealing new sub-populations for what today is classified as one disease
Researchers continue to use HotNet2 to identify novel cancer driver-gene pathways and pan-cancer networks. They are finding numerous applications and questions which can be investigated with their data, including:
- Use within a cancer patient cohort to identify distinct mutation profiles of heterogeneous tumour cell subpopulations to define patient subpopulations for optimising treatment
- Extending analysis to non-coding variants across disease populations
- Integrating diverse ‘Omics data to reveal novel targets and target pathways
- Determining protein-protein interaction networks to analyse gene expression data
- Examining the relationships between genetic changes and clinical phenotypes, including drug responses, in genome-wide association studies (GWAS), among others
Removing challenges to implementing HotNet2 for your data
There are, of course, challenges that present themselves when using a high performing algorithm like HotNet2, including:
- Installation is quite complex as HotNet2 comes with many dependencies.
- To increase the power of analysis, experimental setups need to include as many samples as possible. Unless you have endless resources to spend on sequencing and data generation, most of the time researchers will somehow need to combine disconnected data from various public and private sources.
- Scaling analysis is critical, as the large cohort sizes offer the optimal ability to reveal new findings and will require massive compute resources to smoothly run the algorithm.
If we were living in an ideal world, researchers wanting to use HotNet2 would be able to skip the tedious installation process, run the algorithm in-house over distributed data using federated capabilities, and have immediate access to infinite compute resources to scale analyses as needed. This is particularly important when you are initially evaluating the importance of novel bioinformatics solutions such as HotNet2.
Seamlessly execute your HotNet2 analyses with Lifebit CloudOS
Well, here at Lifebit, we are close to an ideal world… bioinformatically-speaking of course.
We are excited to announce that Lifebit and Medley Genomics have joined forces to streamline the implementation of HotNet2 by making it available to everyone through the Lifebit CloudOS Marketplace.
“Increasingly our customers are applying HotNet2 to define subgroups within their patient disease cohorts and to reveal novel biological pathways. Our partnership with Lifebit enables us to reach countless more researchers across our shared communities – helping them to simplify their work processes and ultimately bring important discoveries to patients faster.” Patrice Milos – Medley Genomics CEO
Importantly, this collaboration removes any challenges, allowing you to derive the value of this important application for your research with precision and speed.
By deploying HotNet2 using Lifebit CloudOS, you will be able to seamlessly execute your analyses, by:
- Skipping the complex installation and getting right into action with HotNet2 – we’ve already done the work for you!
- Uniting distributed data through CloudOS’ federated analysis capabilities – without putting your data at risk by having to transfer it from one place to another.
- Scaling analysis to fit your real-time needs – easily run either a sample-level analysis within your cohort or across different patient cohorts.
- Creating, sharing & collaborating – generate detailed reports, including visualisations for each run, seamlessly share and collaborate with your team members and easily reference results on publications by simple link-share.
Importantly, these capabilities, only offered by Lifebit’s CloudOS system, will enable any company to implement HotNet2 at enterprise scale by automating analysis in their computational environment using the powerful Lifebit CloudOS APIs.
Try it!
Are you curious to see what a cloud-native HotNet2 job looks like? Working with the Medley Genomics team, check out our successful HotNet2 job where we reproduce the pan-cancer network analysis mentioned above using Dr. Raphael’s original code.
Have your own data to test drive? Run the scalable HotNet2 algorithm on the CloudOS platform.
If you are already using HotNet2 on Lifebit’s CloudOS platform, we would love to know what you think! If you’re interested in running our pipelines, contact our Customer Success team below, they would love to help you out!
Featured news and events
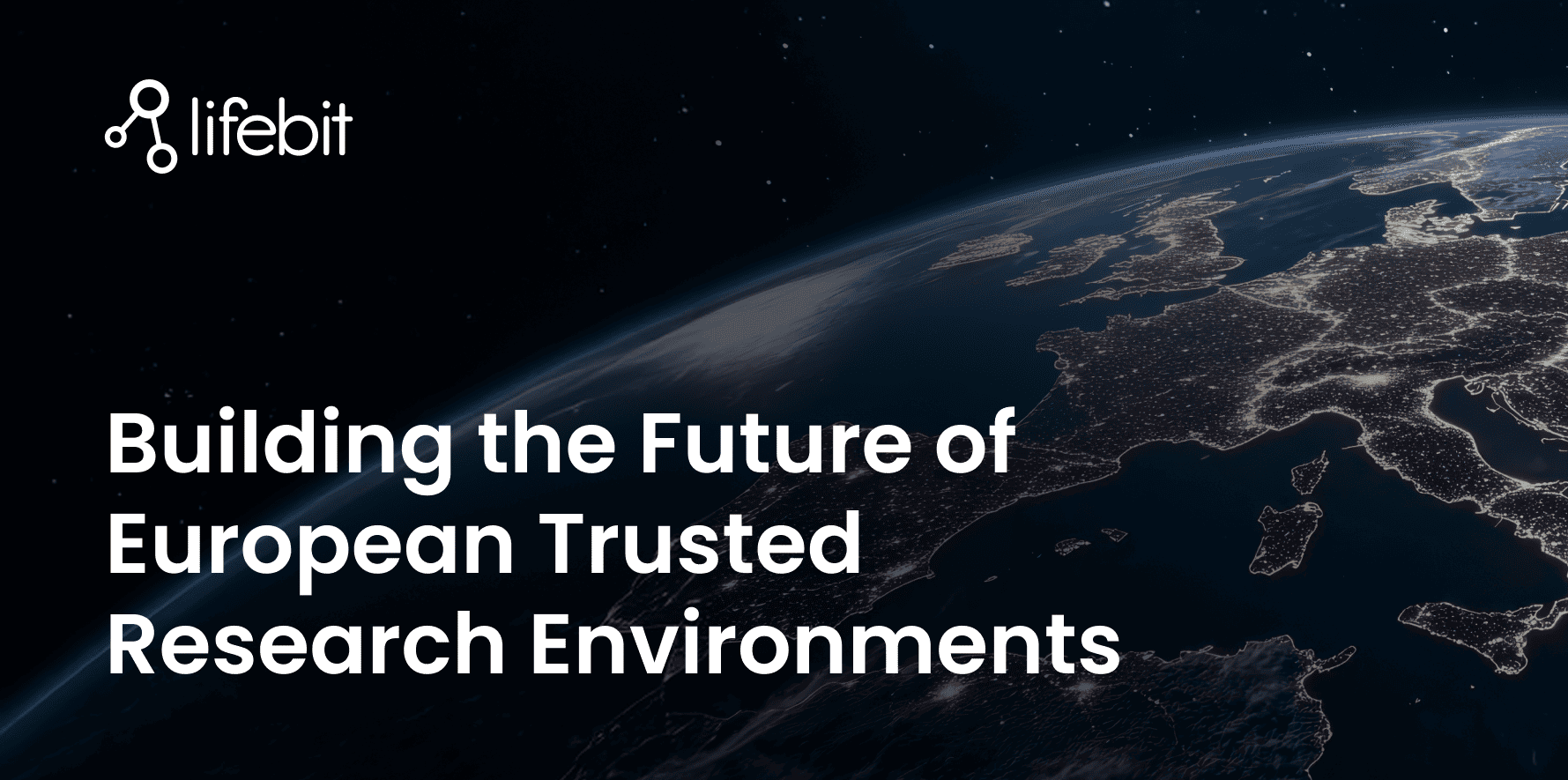
2025-03-26 11:17:46
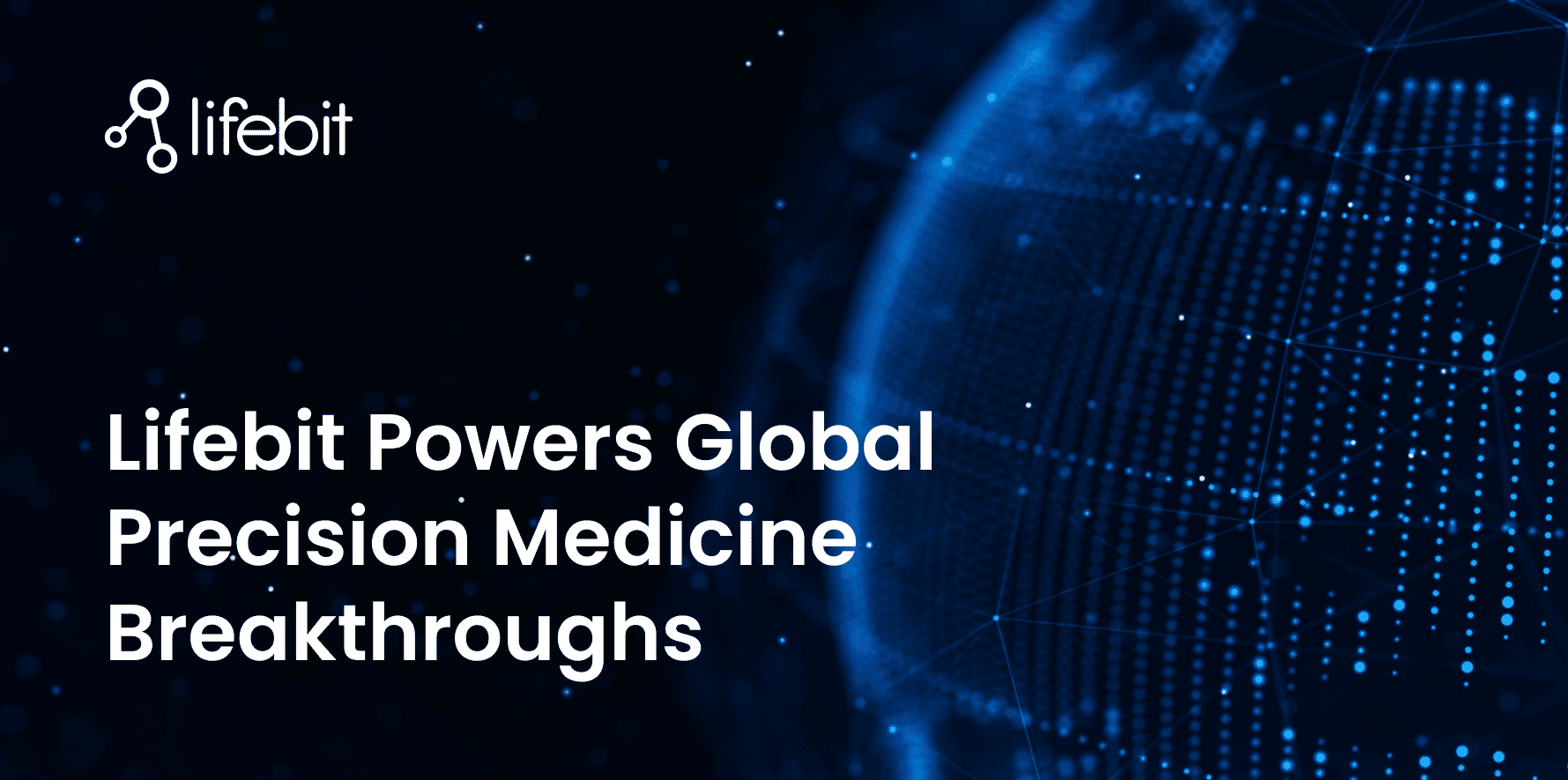
2025-03-14 15:45:18
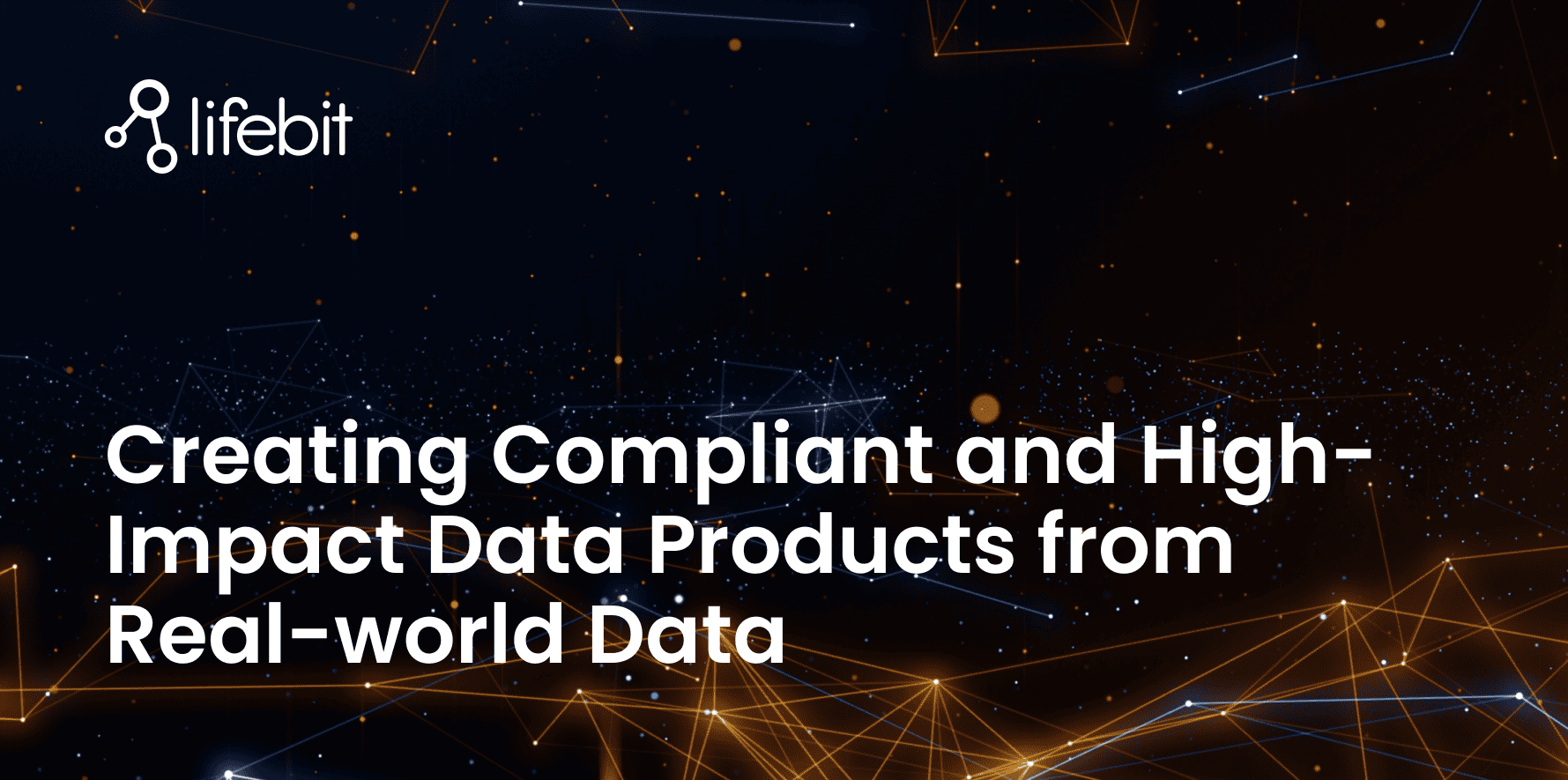
2025-03-05 12:49:53
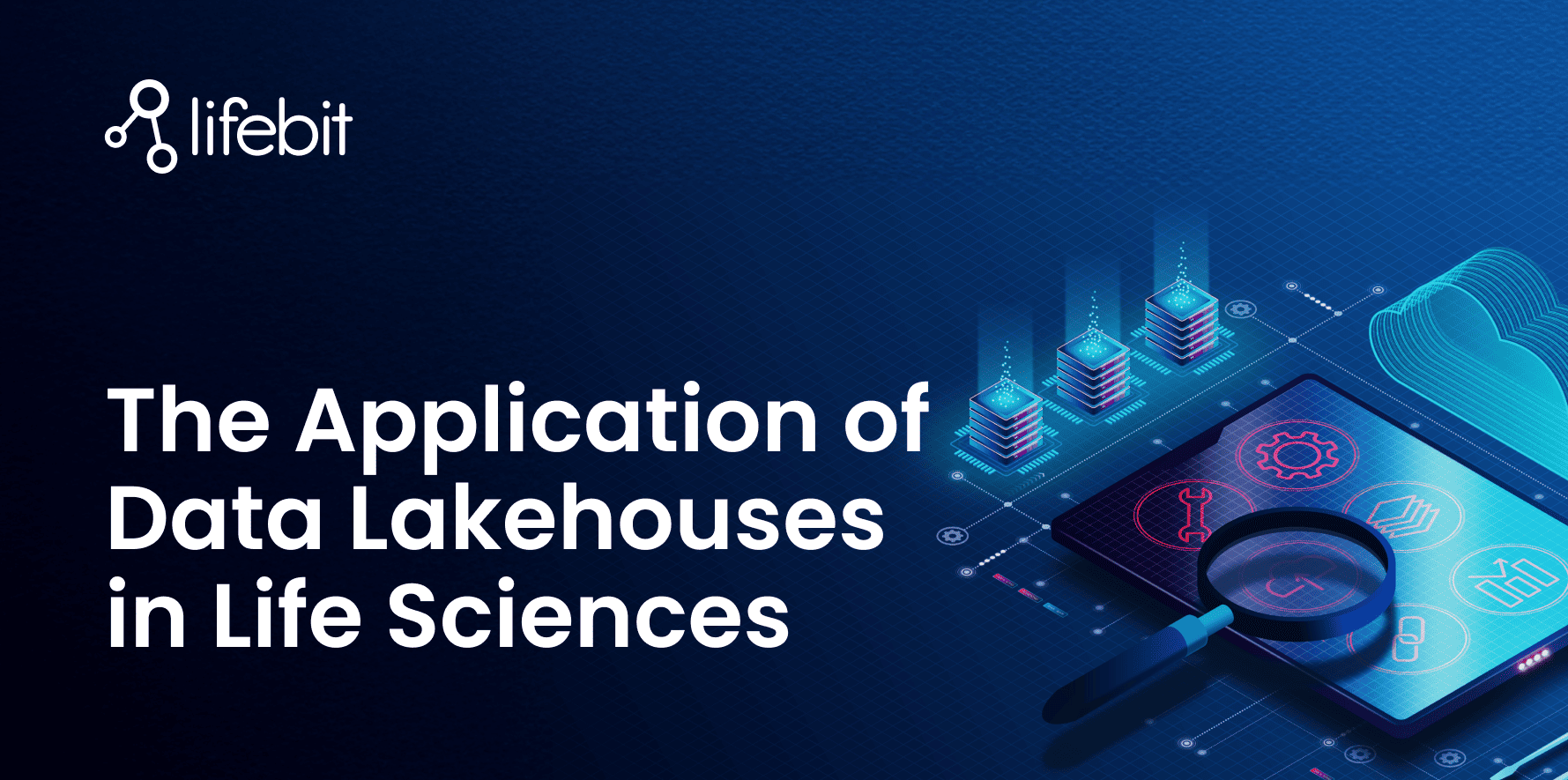
2025-02-27 10:00:00
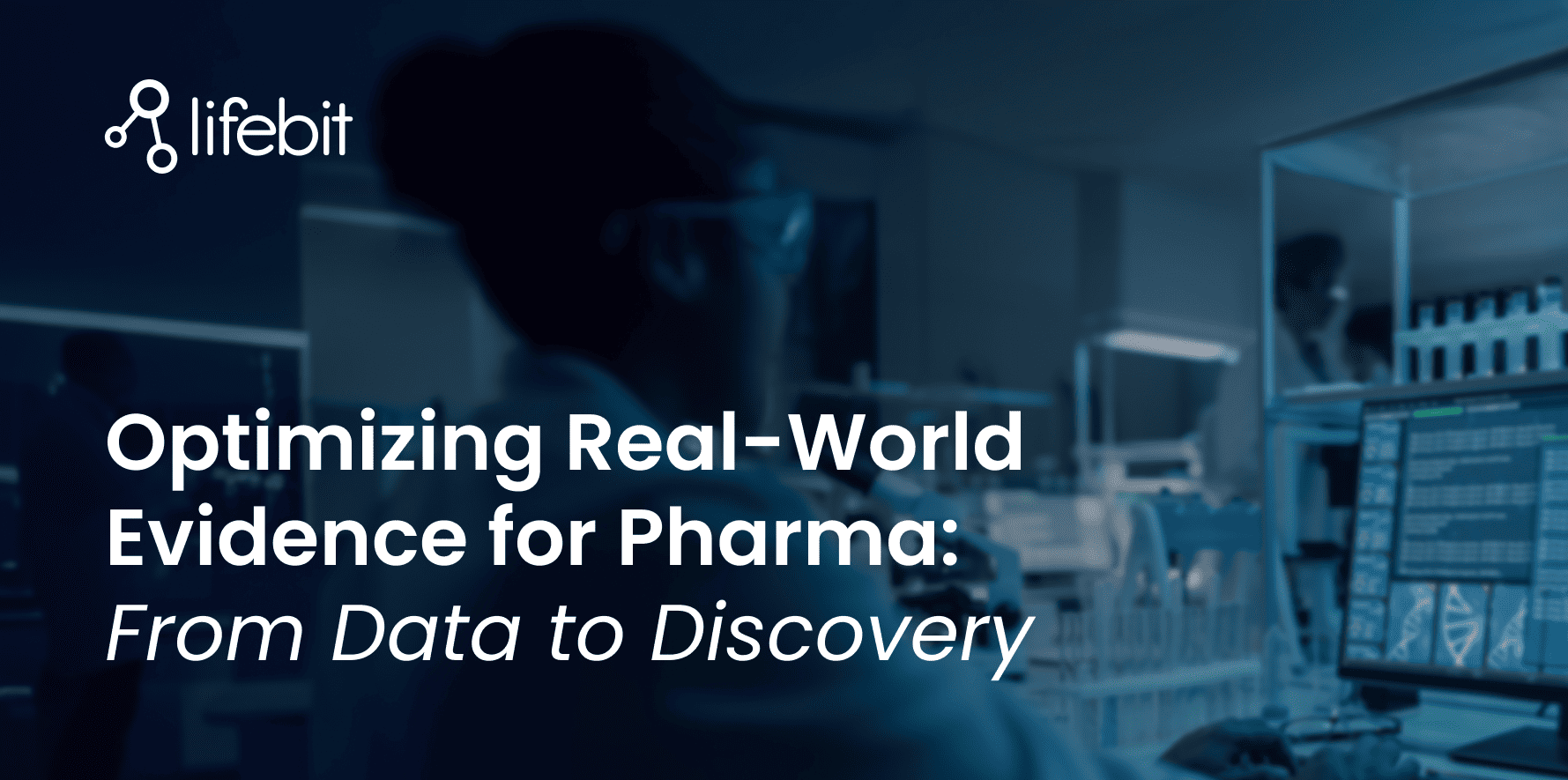
2025-02-19 13:30:24
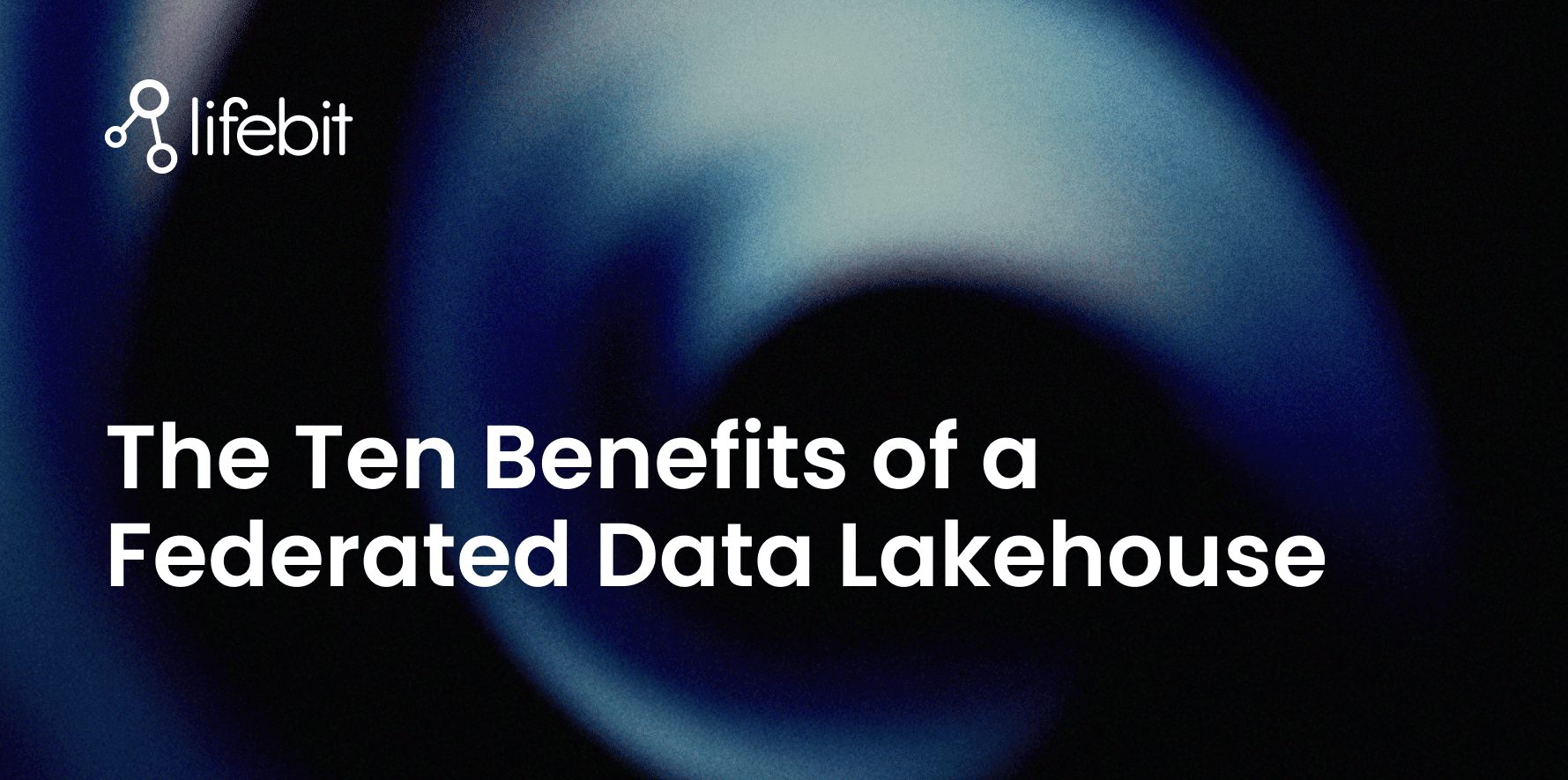
2025-01-30 12:47:38